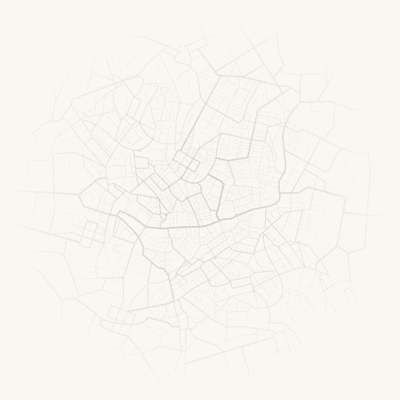
StreetLight Resources
Dive into the world of transportation data
Navigate the ever-evolving world of transportation planning with StreetLight as your trusted guide. Our comprehensive resource hub provides you with the knowledge, connections, and tools you need to make informed decisions and build a more efficient, safe, and sustainable transportation network
Filter
Transportation Climate Impact Index
Download our 2024 U.S. Transportation Climate Impact Index to learn where your city ranks across eight key emissions factors.


Webinars
Traffic Operations Beyond Sensors: Real-time, network-wide dashboard to plan, forecast, monitor

Research Report
The Transportation Mode Share Report: Ranking U.S. counties for active transportation

Case Study
Curbing school zone crashes in the 5th largest U.S. school district

Videos
Improving Intersection Safety With Turning Movement Counts by Time-of-day

Videos
Missouri DOT uses StreetLight to fill data gaps: planning safer lane closures & interchange designs

Videos
Analyzing Hard Braking and Acceleration Events With StreetLight

Videos
Evaluating Corridor Speed Changes and Traffic Calming With Streetlight

Case Study
Boulder reveals effective ways to cut transportation emissions by 80%

Videos
Making School Zones Safer in Clark County, Nevada | Expert Shares What the Data Revealed
Unleash the potential of data: Discover StreetLight
Explore the resources above and don’t hesitate to contact us if you have any questions. We’re here to partner with you in building a smarter transportation future for your community.